An Intro to AI Tech Through Vineyard Integrated Pest Management (IPM)
A beginner's guide to the transformative role Artificial Intelligence is and will continue to play in integrated pest management and vineyard operations.
6/15/20234 min read
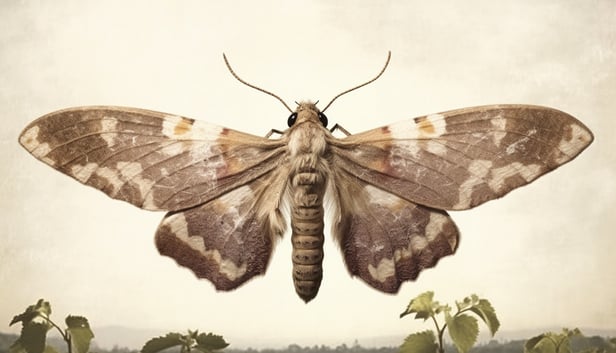
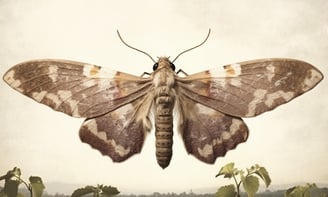
Integrated Pest Management (IPM) in viticulture is a science-based decision-making process that identifies and reduces risks from pests while minimizing economic, environmental, and health risks. One key development enhancing IPM's effectiveness is the application of Artificial Intelligence (AI) technologies. The integration of AI in viticulture is a burgeoning field, offering promise in enhancing decision-making capabilities, improving pest prediction, and optimizing pesticide usage. This essay elucidates the role of AI in supporting IPM for vineyards, delving into the technical facets of machine learning algorithms, image recognition technology, remote sensing, and predictive modeling.
AI and Pest Identification
A fundamental challenge in IPM is the precise identification of pests. Traditional pest scouting methods are time-consuming and depend on the acuity and expertise of the individual scout. AI technologies can streamline this process, offering rapid, efficient, and accurate pest identification.
Machine learning (ML), a subset of AI, involves teaching computers to learn and make decisions from data. Supervised learning, an ML paradigm, can be used for pest identification. This approach involves training a model on a labeled dataset comprising images of pests and non-pests. This labeling is typically performed manually by an expert who assigns each image a label corresponding to its class (pest or non-pest). Once the model is trained, it can be used to classify new, unseen images automatically. One popular algorithm for image classification tasks is the convolutional neural network (CNN), a type of deep learning model that excels in handling image data.
The process of training a CNN involves several steps. First, the input image is passed through a series of convolutional layers. Each layer applies a set of filters to the image, producing a number of feature maps. The features learned by these layers are abstract: while early layers may learn to detect edges, curves, and textures, later layers combine these simpler features to detect complex patterns.
The output from the convolutional layers is flattened into a one-dimensional vector and fed into one or more fully connected layers. These layers learn to map the extracted features to the image labels. During training, the model adjusts the parameters of the filters and the fully connected layers to minimize the difference between its predictions and the true labels. This process involves a method called backpropagation, which uses gradient descent to optimize the model's parameters.
Remote Sensing for Pest Detection
Remote sensing technology has become a valuable tool for pest management in vineyards. This technology allows for data collection over large areas and in real-time, enabling vineyard managers to spot pest hotspots quickly and to intervene effectively. Unmanned aerial vehicles (UAVs), or drones, equipped with multispectral cameras, are frequently used for this purpose.
Multispectral imaging involves capturing image data within specific wavelength ranges across the electromagnetic spectrum. For instance, near-infrared light (NIR) is typically reflected by healthy vegetation, while green light is absorbed. Unhealthy or stressed plants, such as those affected by pests, reflect less NIR light and absorb more visible light. As such, by comparing the amount of visible and NIR light reflected by plants, we can assess their health.
The data collected by the multispectral cameras are processed using machine learning algorithms. These algorithms, trained on a dataset of labeled multispectral images, can classify each pixel of a new image as "pest-infested" or "healthy". By generating a pixel-level classification map, these algorithms allow vineyard managers to visualize the extent and severity of a pest outbreak.
Predictive Modeling for Pest Management
Besides pest identification, AI technologies can also predict pest dynamics, providing vineyard managers with an advanced warning system. Predictive models can be developed using machine learning algorithms to forecast future pest populations based on past population trends and environmental variables.
Various machine learning algorithms, such as random forests, support vector machines, and artificial neural networks, have been used for this purpose. These algorithms learn patterns in historical data, and by leveraging these patterns, they predict future pest populations.
Random forest, for example, is a powerful ensemble learning method that constructs multiple decision trees and aggregates their predictions. Each decision tree splits the data based on a certain condition to predict the target variable. Random forests introduce randomness in two ways: by training each tree on a random subset of the data and by splitting each node based on the best split among a random subset of the features. This randomness makes the model more robust and prevents overfitting.
Optimizing Pesticide Use
Overreliance on chemical pesticides can lead to pesticide resistance, environmental contamination, and harm to non-target organisms. AI can help mitigate these issues by optimizing pesticide use. By accurately identifying pest hotspots and predicting future pest populations, AI enables targeted pesticide application. This approach, known as precision viticulture, not only minimizes pesticide usage but also ensures that pesticides are applied at the most effective time and place.
Moreover, machine learning models can optimize the dosage and timing of pesticide applications. Reinforcement learning, an area of machine learning concerned with how software agents ought to take actions in an environment to maximize the notion of cumulative reward, can be used for this purpose.
AI technologies offer a wealth of opportunities to enhance IPM in vineyards. By integrating AI with traditional IPM strategies, we can revolutionize pest management, making it more efficient, effective, and environmentally friendly. As advances in AI continue at a rapid pace, we can anticipate further innovations that will drive the evolution of IPM.
Although this post provides an overview of the applications of AI in vineyard IPM, it is by no means exhaustive. Each AI technology has its own strengths and limitations, and the choice of technology depends on the specific requirements of the vineyard. As such, vineyard managers should consult with AI experts to design an AI strategy that best meets their needs. Nevertheless, it is clear that AI has the potential to transform vineyard IPM. The marriage of AI and viticulture can usher in a new era of sustainable wine production, preserving our vineyards for future generations while ensuring the continued production of high-quality wines.
Read More: